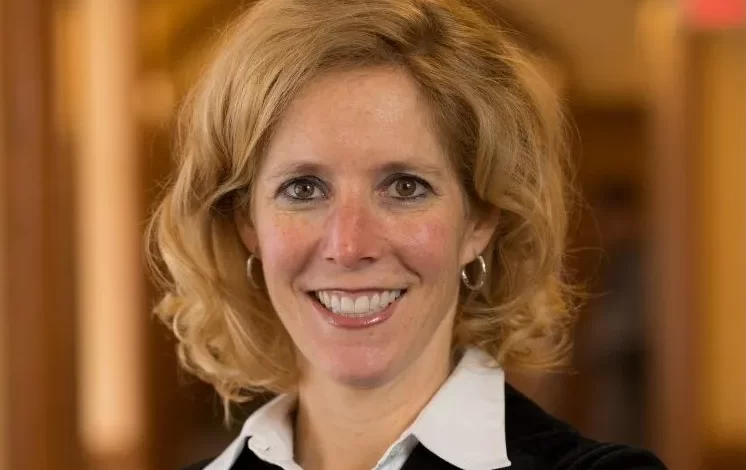
The explosive rise of generative AI is already transforming journalism, finance, and medicine, but it could also have a disruptive influence on politics. For example, asking a chatbot how to navigate a complicated bureaucracy or to help draft a letter to an elected official could bolster civic engagement.
However, that same technology—with its potential to produce disinformation and misinformation at scale—threatens to interfere with democratic representation, undermine democratic accountability, and corrode social and political trust.
This essay analyzes the scope of the threat in each of these spheres and discusses potential guardrails for these misuses, including neural networks used to identify generated content, self-regulation by generative-AI platforms, and greater digital literacy on the part of the public and elites alike.
Just a month after its introduction, ChatGPT, the generative artificial intelligence (AI) chatbot, hit 100-million monthly users, making it the fastest-growing application in history. For context, it took the video-streaming service Netflix, now a household name, three-and-a-half years to reach one-million monthly users.
But unlike Netflix, the meteoric rise of ChatGPT and its potential for good or ill sparked considerable debate. Would students be able to use, or rather misuse, the tool for research or writing? Would it put journalists and coders out of business? Would it “hijack democracy,” as one New York Times op-ed put it, by enabling mass, phony inputs to perhaps influence democratic representation?. And most fundamentally (and apocalyptically), could advances in artificial intelligence actually pose an existential threat to humanity
New technologies raise new questions and concerns of different magnitudes and urgency. For example, the fear that generative AI—artificial intelligence capable of producing new content—poses an existential threat is neither plausibly imminent, nor necessarily plausible. Nick Bostrom’s paperclip scenario, in which a machine programmed to optimize paperclips eliminates everything standing in its way of achieving that goal, is not on the verge of becoming reality.
Whether children or university students use AI tools as shortcuts is a valuable pedagogical debate, but one that should resolve itself as the applications become more seamlessly integrated into search engines.
The employment consequences of generative AI will ultimately be difficult to adjudicate since economies are complex, making it difficult to isolate the net effect of AI-instigated job losses versus industry gains. Yet the potential consequences for democracy are immediate and severe. Generative AI threatens three central pillars of democratic governance: representation, accountability, and, ultimately, the most important currency in a political system—trust.
The most problematic aspect of generative AI is that it hides in plain sight, producing enormous volumes of content that can flood the media landscape, the internet, and political communication with meaningless drivel at best and misinformation at worst.
For government officials, this undermines efforts to understand constituent sentiment, threatening the quality of democratic representation. For voters, it threatens efforts to monitor what elected officials do and the results of their actions, eroding democratic accountability.
A reasonable cognitive prophylactic measure in such a media environment would be to believe nothing, a nihilism that is at odds with vibrant democracy and corrosive to social trust.
As objective reality recedes even further from the media discourse, those voters who do not tune out altogether will likely begin to rely even more heavily on other heuristics, such as partisanship, which will only further exacerbate polarization and stress on democratic institutions.
Threats to Democratic Representation
Democracy, as Robert Dahl wrote in 1972, requires “the continued responsiveness of the government to the preferences of its citizens.” For elected officials to be responsive to the preferences of their constituents, however, they must first be able to discern those preferences. Public-opinion polls—which (at least for now) are mostly immune from manipulation by AI-generated content—afford elected officials one window into their constituents’ preferences. But most citizens lack even basic political knowledge, and levels of policy-specific knowledge are likely lower still.
As such, legislators have strong incentives to be the most responsive to constituents with strongly held views on a specific policy issue and those for whom the issue is highly salient. Written correspondence has long been central to how elected officials keep their finger on the pulse of their districts, particularly to gauge the preferences of those most intensely mobilized on a given issue.
In an era of generative AI, however, the signals sent by the balance of electronic communications about pressing policy issues may be severely misleading. Technological advances now allow malicious actors to generate false “constituent sentiment” at scale by effortlessly creating unique messages taking positions on any side of a myriad of issues. Even with old technology, legislators struggled to discern between human-written and machine-generated communications.
In a field experiment conducted in 2020 in the United States, we composed advocacy letters on six different issues and then used those letters to train what was then the state-of-the-art generative AI model, GPT-3, to write hundreds of left-wing and right-wing advocacy letters.
We sent randomized AI- and human-written letters to 7,200 state legislators, a total of about 35,000 emails. We then compared response rates to the human-written and AI-generated correspondence to assess the extent to which legislators were able to discern (and therefore not respond to) machine-written appeals.
On three issues, the response rates to AI- and human-written messages were statistically indistinguishable. On three other issues, the response rates to AI-generated emails were lower—but only by 2 percent, on average. This suggests that a malicious actor capable of easily generating thousands of unique communications could potentially skew legislators’ perceptions of which issues are most important to their constituents as well as how constituents feel about any given issue.
In the same way, generative AI could strike a double blow against the quality of democratic representation by rendering obsolete the public-comment process through which citizens can seek to influence the actions of the regulatory state.
Legislators necessarily write statutes in broad brushstrokes, granting administrative agencies considerable discretion not only to resolve technical questions requiring substantive expertise (e.g., specifying permissible levels of pollutants in the air and water), but also to make broader judgements about values (e.g., the acceptable tradeoffs between protecting public health and not unduly restricting economic growth).
Moreover, in an era of intense partisan polarization and frequent legislative gridlock on pressing policy priorities, U.S. presidents have increasingly sought to advance their policy agendas through administrative rulemaking.
Moving the locus of policymaking authority from elected representatives to unelected bureaucrats raises concerns of a democratic deficit. The U.S. Supreme Court raised such concerns in West Virginia v. EPA (2022), articulating and codifying the major questions doctrine, which holds that agencies do not have authority to effect major changes in policy absent clear statutory authorization from Congress.
The Court may go even further in the pending Loper Bright Enterprises v. Raimondo case and overturn the Chevron doctrine, which has given agencies broad latitude to interpret ambiguous congressional statutes for nearly three decades, thus further tightening the constraints on policy change through the regulatory process.
Not everyone agrees that the regulatory process is undemocratic, however. Some scholars argue that the guaranteed opportunities for public participation and transparency during the public-notice and comment period are “refreshingly democratic,” and extol the process as “democratically accountable, especially in the sense that decision-making is kept above board and equal access is provided to all.” Moreover, the advent of the U.S. government’s electronic-rulemaking (e-rulemaking) program in 2002 promised to “enhance public participation . . . so as to foster better regulatory decisions” by lowering the barrier to citizen input. Of course, public comments have always skewed, often heavily, toward interests with the most at stake in the outcome of a proposed rule, and despite lowering the barriers to engagement, e-rulemaking did not alter this fundamental reality.
Despite its flaws, the direct and open engagement of the public in the rulemaking process helped to bolster the democratic legitimacy of policy change through bureaucratic action. But the ability of malicious actors to use generative AI to flood e-rulemaking platforms with limitless unique comments advancing a particular agenda could make it all but impossible for agencies to learn about genuine public preferences.
An early (and unsuccessful) test case arose in 2017, when bots flooded the Federal Communications Commission with more than eight-million comments advocating repeal of net neutrality during the open comment period on proposed changes to the rules.
This “astroturfing” was detected, however, because more than 90 percent of those comments were not unique, indicating a coordinated effort to mislead rather than genuine grassroots support for repeal. Contemporary advances in AI technology can easily overcome this limitation, rendering it exceedingly difficult for agencies to detect which comments genuinely represent the preferences of interested stakeholders.
Threats to Democratic Accountability
A healthy democracy also requires that citizens be able to hold government officials accountable for their actions—most notably, through free and fair elections. For ballot-box accountability to be effective, however, voters must have access to information about the actions taken in their name by their representatives.
Concerns that partisan bias in the mass media, upon which voters have long relied for political information, could affect election outcomes are longstanding, but generative AI poses a far greater threat to electoral integrity.
As is widely known, foreign actors exploited a range of new technologies in a coordinated effort to influence the 2016 U.S. presidential election. A 2018 Senate Intelligence Committee report stated:
Masquerading as Americans, these (Russian) operatives used targeted advertisements, intentionally falsified news articles, self-generated content, and social media platform tools to interact with and attempt to deceive tens of millions of social media users in the United States.
This campaign sought to polarize Americans on the basis of societal, ideological, and racial differences, provoked real world events, and was part of a foreign government’s covert support of Russia’s favored candidate in the U.S. presidential election.
While unprecedented in scope and scale, several flaws in the influence campaign may have limited its impact. The Russian operatives’ social-media posts had subtle but noticeable grammatical errors that a native speaker would not make, such as a misplaced or missing article—telltale signs that the posts were fake.
ChatGPT, however, makes every user the equivalent of a native speaker. This technology is already being used to create entire spam sites and to flood sites with fake reviews. The tech website The Verge flagged a job seeking an “AI editor” who could generate “200 to 250 articles per week,” clearly implying that the work would be done via generative AI tools that can churn out mass quantities of content in fluent English at the click of the editor’s “regenerate” button.
The potential political applications are myriad. Recent research shows that AI-generated propaganda is just as believable as propaganda written by humans. This, combined with new capacities for microtargeting, could revolutionize disinformation campaigns, rendering them far more effective than the efforts to influence the 2016 election.
A steady stream of targeted misinformation could skew how voters perceive the actions and performance of elected officials to such a degree that elections cease to provide a genuine mechanism of accountability since the premise of what people are voting on is itself factually dubious.
Threats to Democratic Trust
Advances in generative AI could allow malicious actors to produce misinformation, including content microtargeted to appeal to specific demographics and even individuals, at scale. The proliferation of social-media platforms allows the effortless dissemination of misinformation, including its efficient channeling to specific constituencies.
Research suggests that although readers across the political spectrum cannot distinguish between a range of human-made and AI-generated content (finding it all plausible), misinformation will not necessarily change readers’ minds. Political persuasion is difficult, especially in a polarized political landscape. Individual views tend to be fairly entrenched, and there is little that can change people’s prior sentiments.
The risk is that as inauthentic content—text, images, and video—proliferates online, people simply might not know what to believe and will therefore distrust the entire information ecosystem.
Trust in media is already low, and the proliferation of tools that can generate inauthentic content will erode that trust even more. This, in turn, could further undermine perilously low levels of trust in government. Social trust is essential glue that holds together democratic societies.
It fuels civic engagement and political participation, bolsters confidence in political institutions, and promotes respect for democratic values, an important bulwark against democratic backsliding and authoritarianism.
Trust operates in multiple directions. For political elites, responsiveness requires a trust that the messages they receive legitimately represent constituent preferences and not a coordinated campaign to misrepresent public sentiment for the sake of advancing a particular viewpoint.
Cases of “astroturfing” are nothing new in politics, with examples in the United States dating back at least to the 1950s. However, advances in AI threaten to make such efforts ubiquitous and more difficult to detect.
For citizens, trust can motivate political participation and engagement, and encourage resistance against threats to democratic institutions and practices. The dramatic decline in Americans’ trust in government over the past half century is among the most documented developments in U.S. politics.
While many factors have contributed to this erosion, trust in the media and trust in government are intimately linked. Bombarding citizens with AI-generated content of dubious veracity could seriously threaten confidence in the media, with severe consequences for trust in the government.
Disclaimer: Ahotoronline.com is not liable for any damages resulting from the use of this information Journal of democracy